In this article you will learn about sampling bias, its types, examples. Moreover, this article talks about sampling bias in probability and non-probability sampling, main causes of sampling bias, and how to avoid it. In the end you will get to know about sampling bias in research, survey and psychology.
Imagine conducting a study on how people learn on mobile devices, but the participants are all solely computer users; the results would obviously be meaningless for the research we are attempting.
Sampling bias is a form of inaccuracy that happens when a research study is conducted with a poor selection of participants; it can be avoided by constantly choosing individuals at random and from various situations.
Sampling Bias
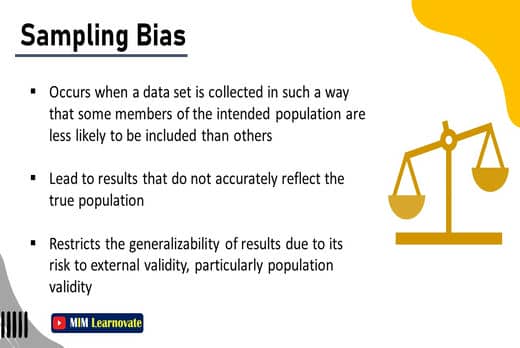
In statistics, sampling bias is a type of bias that occurs when a data set is collected in such a way that some members of the intended population are less likely to be included than others. This can lead to results that do not accurately reflect the true population.
If not accounted for, sampling bias can lead to incorrect conclusions about the population. Sampling bias restricts the generalizability of results due to its risk to external validity, particularly population validity. In other words, the only groups to whom results from biased samples can be generalized are those with similar characteristics.
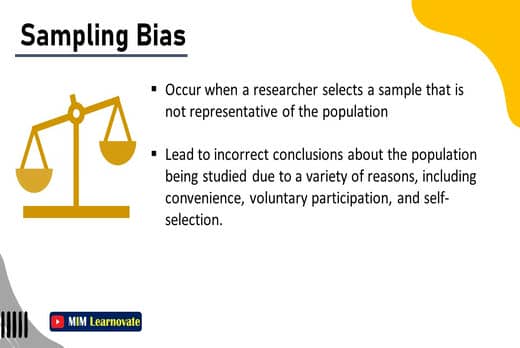
When conducting research, it’s important to be aware of sampling bias. This is a type of error that can occur when a researcher selects a sample that is not representative of the population. Sampling bias can lead to incorrect conclusions about the population being studied.
This can happen for a variety of reasons, including convenience, voluntary participation, and self-selection.
Example of Sampling Bias
If a study only includes people who are willing to participate, it may not be representative of the general population. This could lead the researcher to believe that the findings are applicable to everyone, when in reality they only apply to those who were willing to take part in the study.
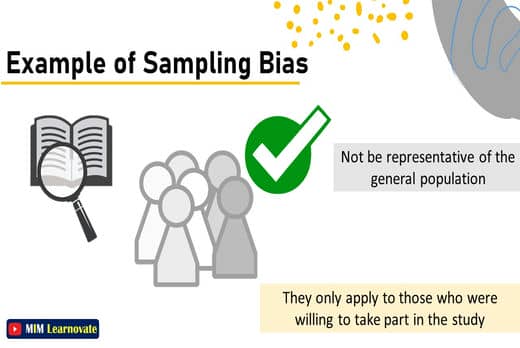
Causes of Sampling Bias
Sampling bias can result from your decision on the research design or data gathering technique. Both probability and non-probability sampling can be subject to this kind of research bias.
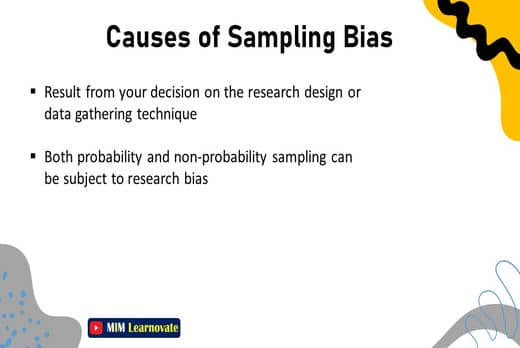
Sampling Bias in Non-Probability Samples
The selection of a non-probability sample is made using non-random criteria. For instance, individuals in a convenience sample are chosen based on their accessibility and availability.
Non-probability sampling frequently yields biased samples because certain population members have a higher likelihood of inclusion than others.
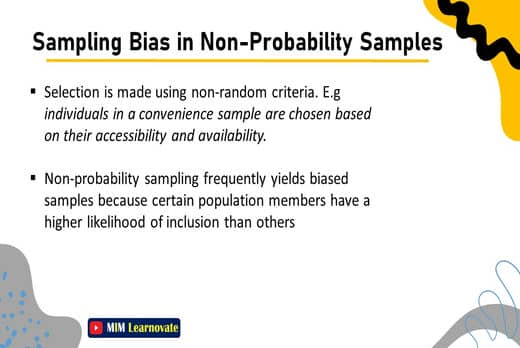
Example of Sampling Bias in Non-Probability Samples
You wish to research how well-liked plant-based foods are among your university’s undergrads. You distribute a survey to all students taking Introduction to Psychology courses at your university out of convenience. They all finish it to receive course credit.
This convenience sample is not representative of your target population because it was chosen at random. Participants in this course might lean more liberally and favor plant-based diets than other students at your university.
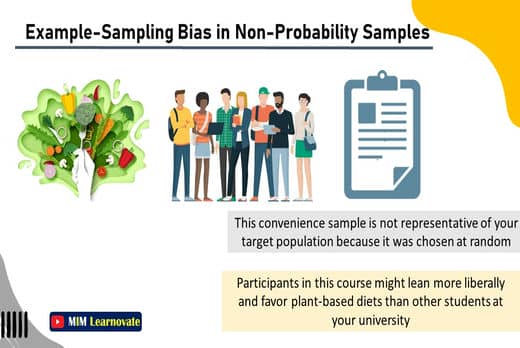
Sampling Bias in Probability Samples
Every member of the population has a known chance of getting chosen in probability sampling. For instance, you can choose a straightforward random sample from your population using a random number generator.
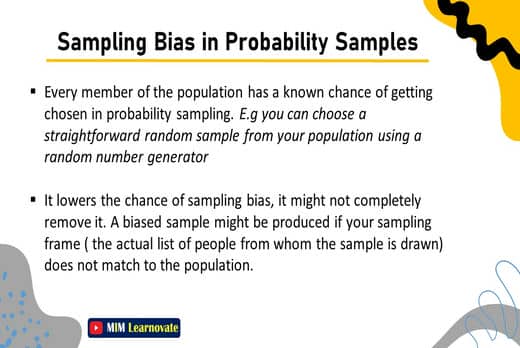
Although this method lowers the chance of sampling bias, it might not completely remove it. A biased sample might be produced if your sampling frame ( the actual list of people from whom the sample is drawn) does not match to the population.
Example of Sampling Bias in Probability Samples
Using a straightforward random sample, you want to examine the levels of procrastination and burnout among graduate students at your university. Using a random number generator, you choose 130 numbers and assign a number between 1 and 2000 to each student in the database of research participants. Despite using a random sample, not all of the graduate students at your university who were your target audience had a chance of getting chosen. Anyone who did not opt-in to be contacted about participating in research is not included in your sample. This could skew your sample in favor of those with lower levels of burnout and more openness to research participation.

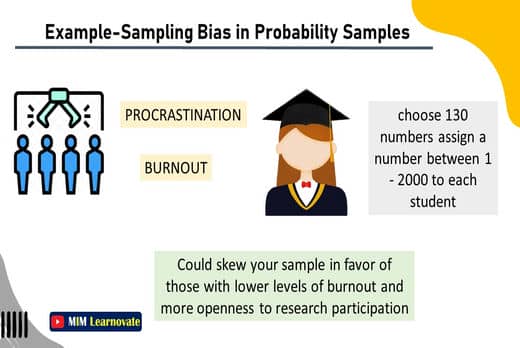
Types of Sampling Bias
There are several types of sampling bias that can occur when conducting research. Some of the more common types include:
- Self-selection Bias
- Non response Bias
- Under coverage Bias
- Observer Bias
- Survivorship Bias
- Healthy User Bias
- Recall Bias
- Pre-screening or advertising Bias
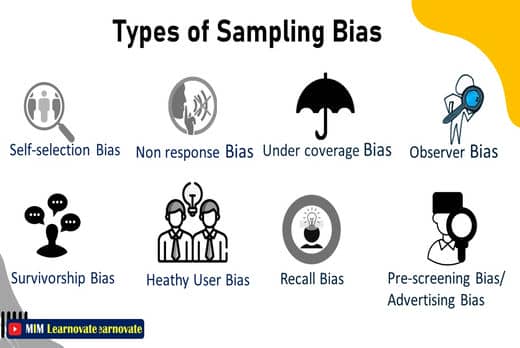
Self-selection bias
One common cause is self-selection bias, which occurs when people who are more likely to participate in a study are also more likely to have certain characteristics that the researcher is interested in. participants in a study choose to participate based on their own personal characteristics.
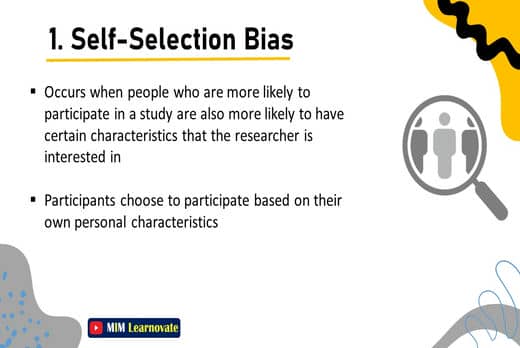
Example of Self-selection bias
If a researcher is studying the effects of a new medication on depression, people who are more likely to participate in the study may be those who are already taking other medications for their depression and are therefore more likely to have severe symptoms.
If a study is looking at the effects of a new weight loss drug, those who are most likely to participate are those who are already interested in losing weight. The results of the study are biased because those who do not participate may have different results.
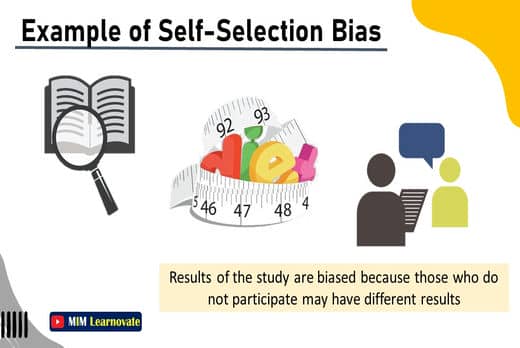
Non response Bias
Another common cause of sampling bias is response bias, which can occur when people with certain characteristics are more or less likely to respond to a researcher’s questions.
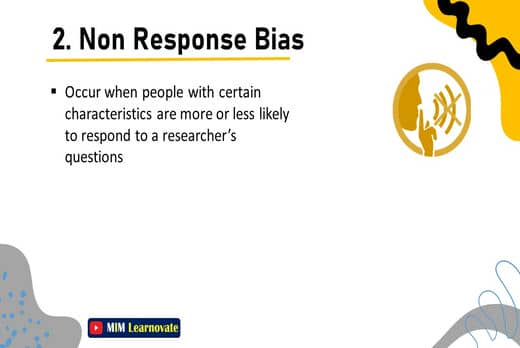
Example of Non response Bias
If a researcher is studying attitudes towards abortion, people who are opposed to abortion may be less likely to respond to the survey than those who support it.
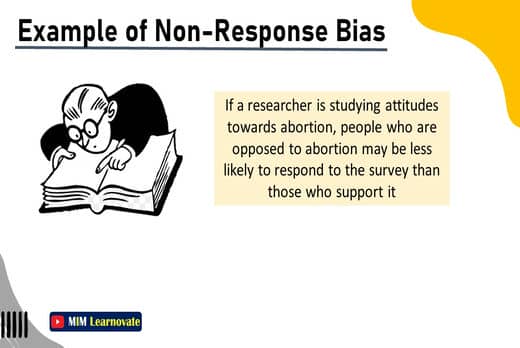
Under coverage Bias
The sample does not fully represent all members of the population. Main cause of under coverage or an insufficient representation of the population is when convenience sampling is used to gather data primarily from respondents who were conveniently accessible,
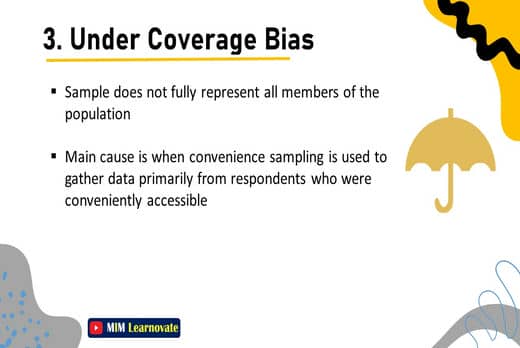
Example of Under coverage Bias
Online broad nationwide surveys may not reach populations like the elderly and lower-income households that have restricted internet access.
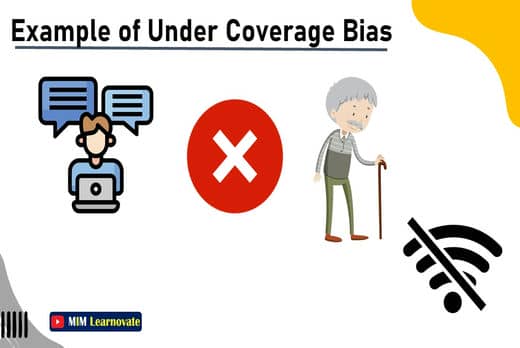
Researchers use convenience sampling to conduct a survey inside a grocery store to determine the impact of a new traffic legislation in a city. The following categories are very likely to be under coverage in the study: ✔Those who dislike going to the grocery store
✔Those without access to a vehicle for grocery store
✔Those who would rather shop at another grocery store
Observer bias
When researchers unconsciously put their expectations onto the research, observer bias occurs. It happens as a result of the practice of examining only a particular set of statistics or influencing interview participants. A good survey design can completely be controlled by the researcher and lessen this bias.
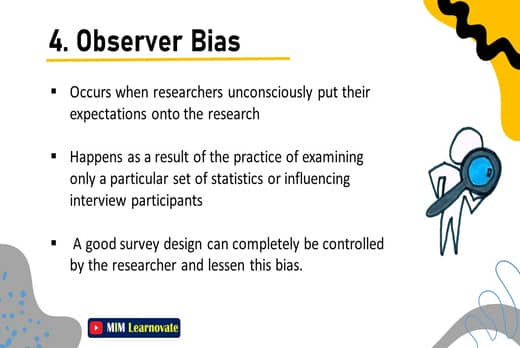
Survivorship Bias
Another prevalent sort of sample bias is survival bias, in which the researcher only pays attention to samples that meet the criterion for selection and ignores those that did not. The issue with survivorship bias is that it produces extremely positive results, which prevents the researcher from getting the full picture.
The results are biased because the opinions of the variables that did not meet the criterion are disregarded. Lack of visibility causes a logical mistake and skews the outcome.
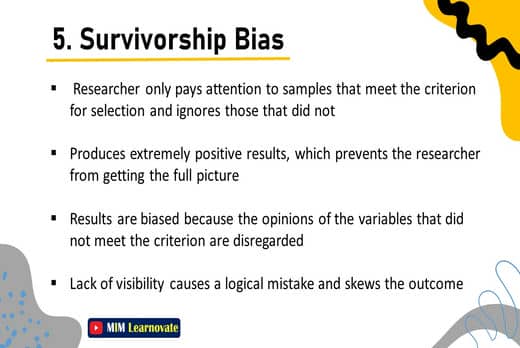
Example of Survivorship Bias
Organizations that have failed and are no longer in existence may not be taken into account when analyzing business success in a particular industry. Due to survivorship bias, the results could appear favorable, but they might not fully reflect the entire sector.
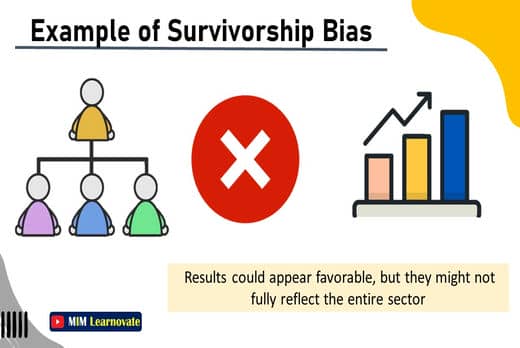
Healthy User
In epidemiologic studies and in medicine, this kind of sample bias is typical. Healthy user sampling bias simply refers to the fact that volunteers for clinical trials and medical research are frequently very different from the population at large.
These people frequently have better health and are more active than the rest of the study population. As a result, you wind up studying individuals who are in good enough health to participate in an activity rather than individuals who would partake in the activity if they were in good health.
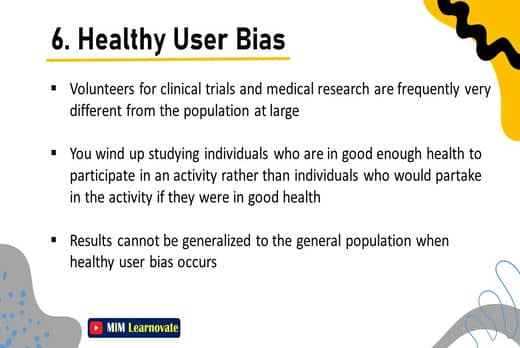
The results of a study or piece of research cannot be generalized to the general population when healthy user bias occurs. Encourage various persons in the research population to take part in your study as a means to counter the healthy user effect.
Example of Healthy User
A preventative intervention sample is healthier than the majority of the population in terms of diet, physical activity levels, liquor absence, and smoking avoidance. Instead of just the therapy itself, the experimental results can represent the outcome of the treatment interacting with various sample characteristics.
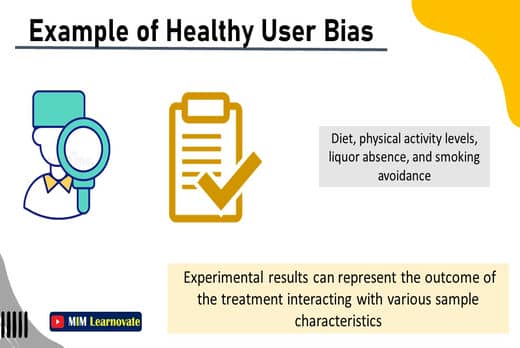
Recall Bias
An inaccuracy that frequently occurs in survey and interview settings is recall bias. This occurs when a respondent is forgetful. The fact that humans naturally have a selective memory means that it is not a matter of having a good or bad memory. Recall bias cannot be completely eliminated; you can only accept it as a common data collection error.
By gathering data when a respondent’s memory is still fresh, one can prevent some of the effects of recall bias.
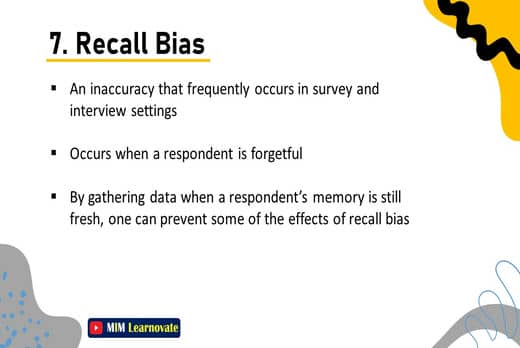
Pre-screening or advertising Bias
When the selection procedure used in a study yields a sample that is not a good reflection of the population, it is called pre-screening or advertisement bias. Some groups may be prevented from participating in a study by its selection methods.
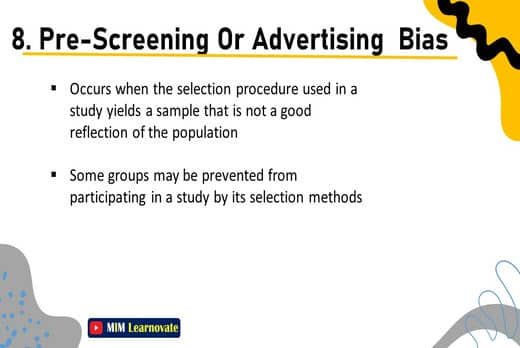
While there may be excellent reasons to pre-screen study participants, doing so might significantly skew your results and the investigative process as a whole. This is due to the possibility that you may choose individuals who have comparable traits that will influence the outcome.
Example of Pre-screening or advertising Bias
You might find that the sample you use for testing a novel sleep therapy is more motivated than the general population to change their sleeping patterns. As a result, regardless of the results of your therapy, individuals might have been more likely to improve their sleeping patterns.
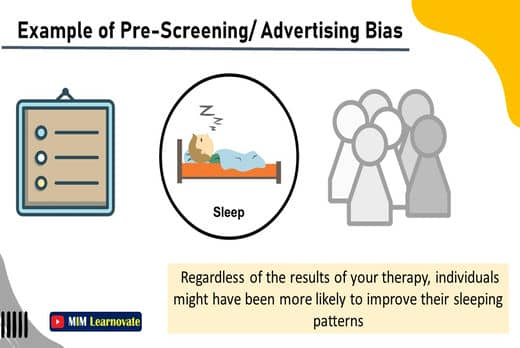
Exclusion Bias
This bias occurs from excluding out particular groups from your sample, such as those who have recently migrated into the study area. The validity of your study may be impacted if you exclude individuals or volunteers who relocate outside the relevant study area.
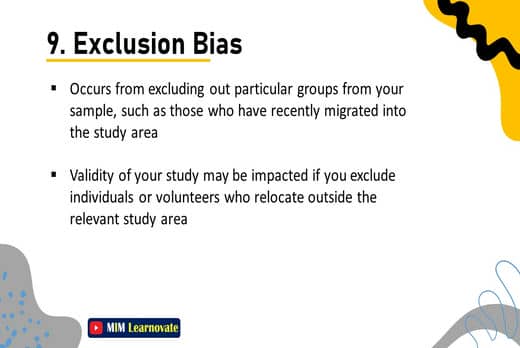
Steps to avoid Sampling Bias
- Define a sampling frame and a target population
- Match the sample frame as closely as possible to the target population.
- Make sure that your sampling frame and target population are compatible.
- Make sure that everyone has an equal chance of being selected for your study.
- Make online surveys as brief and user-friendly as you can.
- Check up on people who don’t respond
- Create survey goals.
- Give responders a fair opportunity to participate
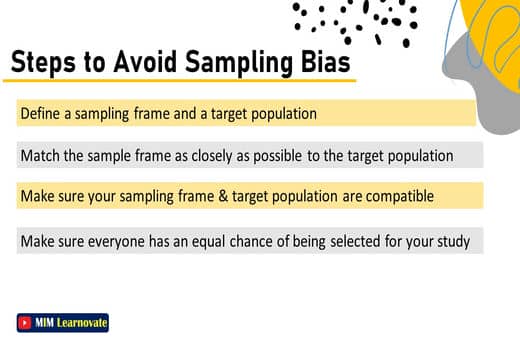
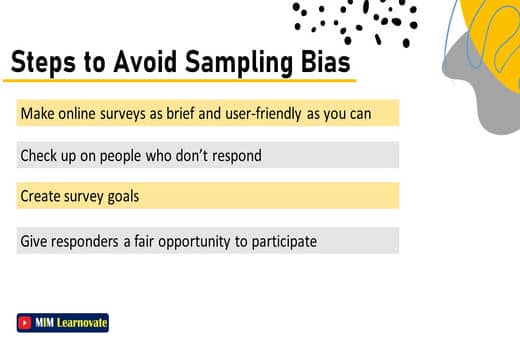
Oversampling to avoid Bias
One way to avoid bias when creating a sample is to oversample. This means that you take extra care to include groups that are often underrepresented in surveys or research.
Example of Oversampling
If you’re studying the effects of a new medication, you might want to oversample people of different ages, genders, and races to make sure that your results are representative of the population as a whole.
To eliminate any sampling bias, responses from oversampled groups are weighted according to their actual share of the population after all data has been gathered. There are several benefits to oversampling.
Benefits of Oversampling
✔First, it can help improve the accuracy of your results by reducing variability.
✔Second, it can help ensure that your results are generalizable to the population of interest.
✔ Third, it can allow you to study rare groups that would be otherwise difficult to identify and recruit for participation in your research.
Sampling Bias in Surveys
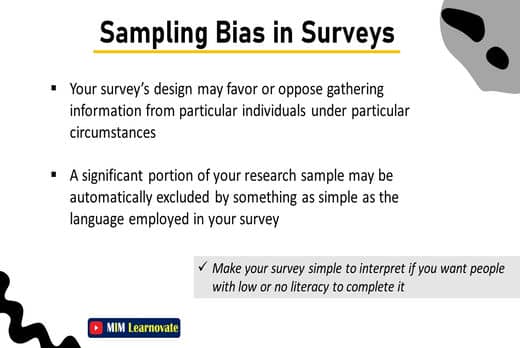
The sort of questions you include in your survey as well as your survey design both have the potential to introduce sampling bias. Your survey’s design may occasionally favor or oppose gathering information from particular populations or individuals under particular circumstances.
A significant portion of your research sample may be automatically excluded by something as simple as the language employed in your survey.
✔ Make your survey simple to interpret if you want people with low or no literacy to complete it.
Example of Sampling Bias in Surveys
Surveys can contain sampling bias in a number of ways. Excluding teens and young adults who are poor or illiterate will biased the results of a survey. While measuring smoking among teenagers and young people.
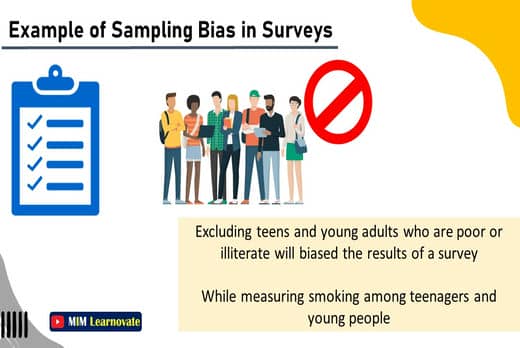
Sampling Bias in Psychology
Sampling bias, particularly health user bias and self-selection bias, can have an impact on research and clinical trials in psychology. When this occurs, the process’s internal validity is severely compromised, which increases the likelihood of many errors.
Work on collecting information from a research population that is well diversified to lessen sampling bias in psychology. You can build a sample frame, which is a list of people from whom research data will be collected. After that match the sampling frame to the target population.
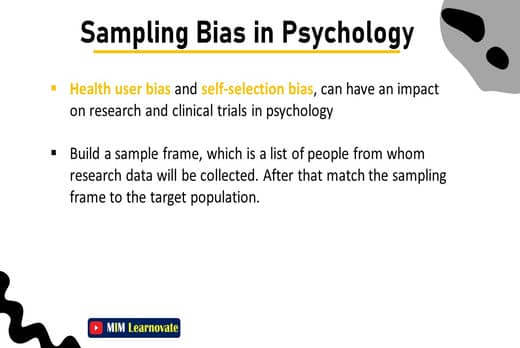
Example of Sampling Bias in Psychology
Think of a study that tries to comprehend the mental health of people in a specific group. The researcher attempts to obtain volunteers for the study in order to collect the needed data. When persons with good or excellent mental health volunteer, this can result in health user bias. Findings of this research might not be a true reflection of what is available in the community.
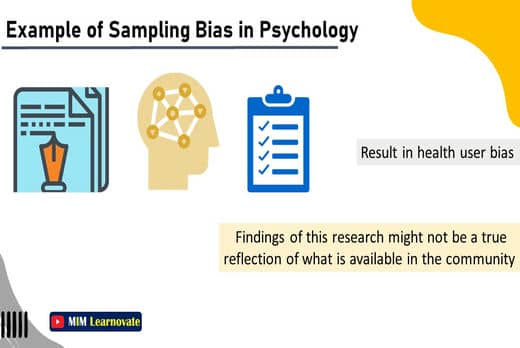
Sampling Bias in Research
A sampling technique used in research is biased if it favors some research findings over others. As we’ve already mentioned, sampling bias in research can happen even when you choose your samples at random. It is not impossible to avoid it, despite this.
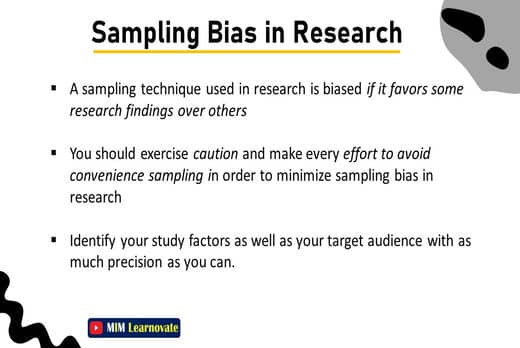
You should exercise caution and make every effort to avoid convenience sampling in order to minimize sampling bias in research. Identify your study factors as well as your target audience with as much precision as you can.
Example of Sampling Bias in Research
An group decides to do study to learn the causes of voter apathy in a particular area. The researcher chooses to conduct a survey in a shopping center in the area to collect the necessary data.
This sample technique already leaves out a number of groups of eligible voters in the area. It doesn’t include those who can’t afford to shop at the shopping centers. Also those who won’t even be there when the survey is conducted. Findings of convenience sampling do not accurately reflect the opinions of the general public when it comes to voter apathy. As a result, the research’s conclusions will be seriously defective and cannot be regarded as reliable.
1 Comment
I haven¦t checked in here for some time as I thought it was getting boring, but the last several posts are great quality so I guess I¦ll add you back to my daily bloglist. You deserve it my friend 🙂