Quasi-experimental designs are a type of research design that is often used in the social sciences.
A quasi-experimental design does not involve random assignment. Instead, participants are assigned to groups based on existing characteristics, such as gender or treatment history.
A quasi-experimental design is a type of experimental design that does not meet all the requirements for a true experiment. Specifically, quasi-experiments lack random assignment to conditions. A quasi-experiment is an observational study that seeks to measure the effect of an intervention by comparing outcomes under conditions that are as similar as possible.
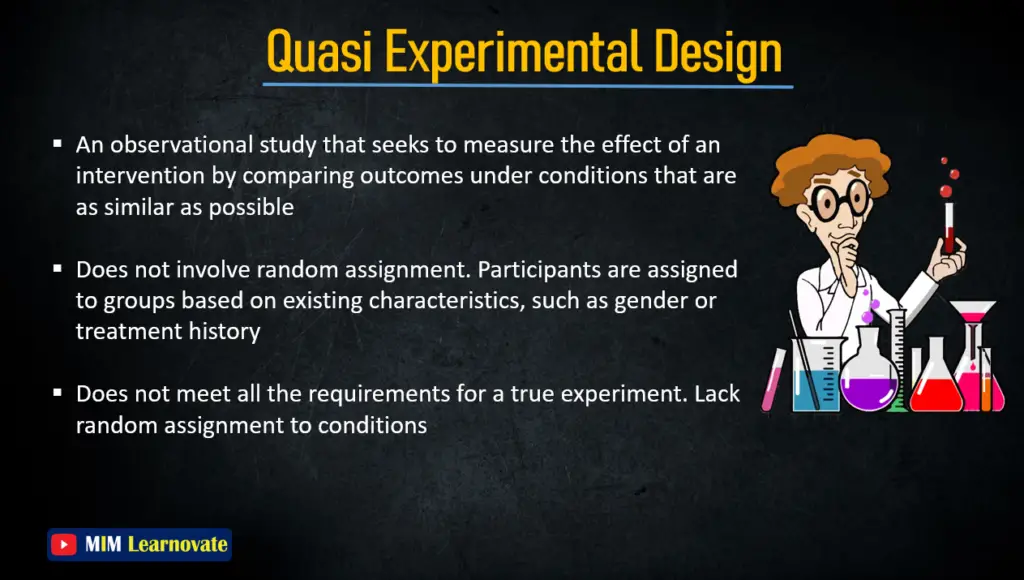
Quasi-experimental designs are often used in situations where random assignment is not possible or practical. Despite not meeting all the criteria for a true experiment, quasi-experimental designs can still be useful in providing evidence for causal relationships.
Read More: Measurement of Scale | Examples | PPT
Quasi-experimental designs are often used when it is not ethical to randomly assign participants to groups, such as when investigating the long-term effects of a treatment.
Example of Quasi-Experimental Design
1- One example of a quasi-experimental design is a before-and-after study. In a before-and-after study, the researcher observes the participants in the treatment group before and after they receive the treatment.
The researcher also observes a control group that does not receive the treatment. The researcher then compares the two groups to see if there is a difference in the outcome variable(s) of interest.
2- Another example of a quasi-experimental design is an interrupted time series design.
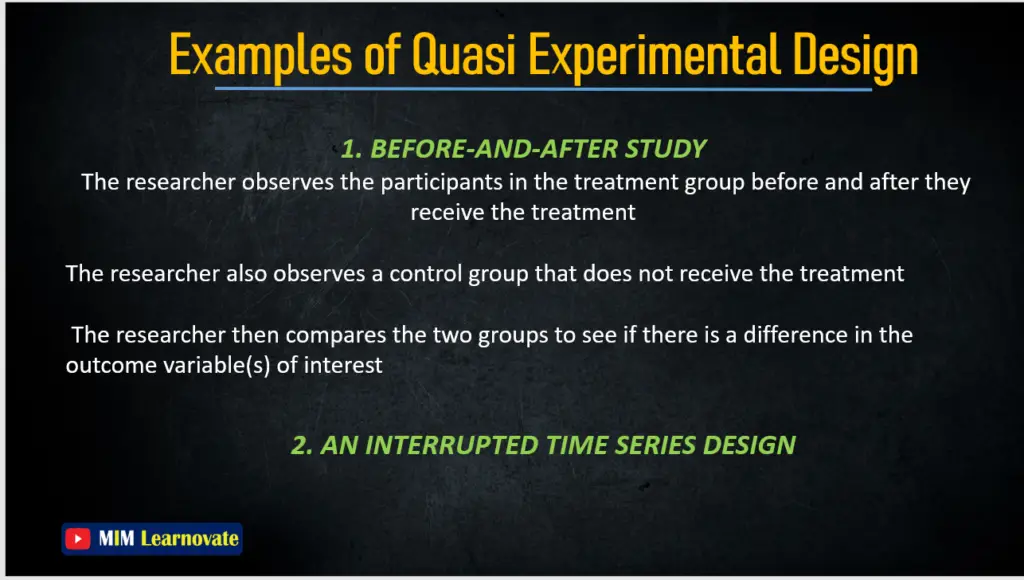
Quasi-Experimental Design vs Experimental Design
This type of design is similar to experimental designs, but there is one key difference.
In quasi-experimental designs, the researcher does not have complete control over the assignment of subjects to treatment and control groups. This means that there is the potential for confounding variables to impact the results of the study.
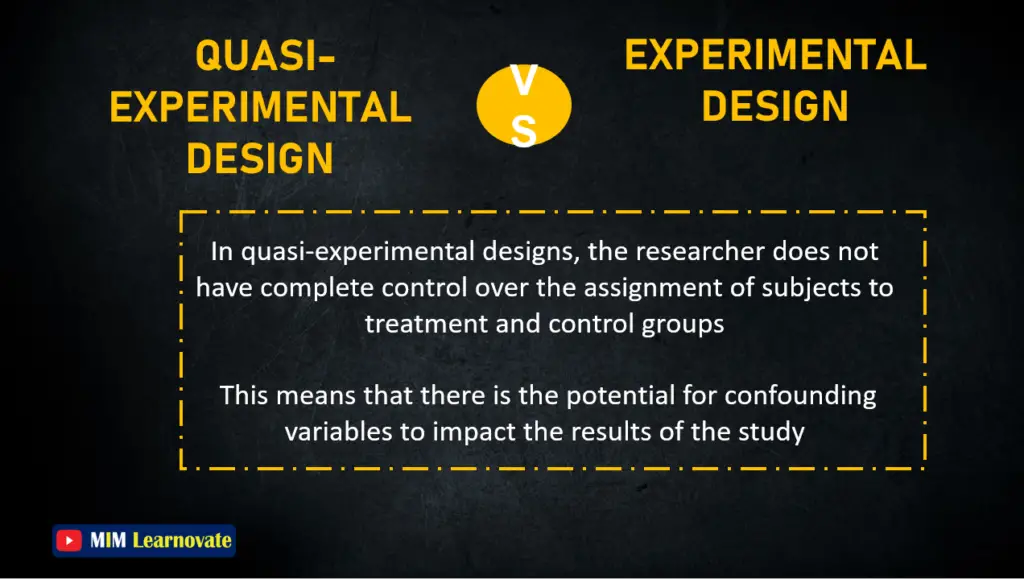
Quasi-Experimental Design vs True experiment
Quasi-experiments differ from true experiments in that they do not randomize subjects to different conditions, but they can still be useful for measuring the causal effects of an intervention
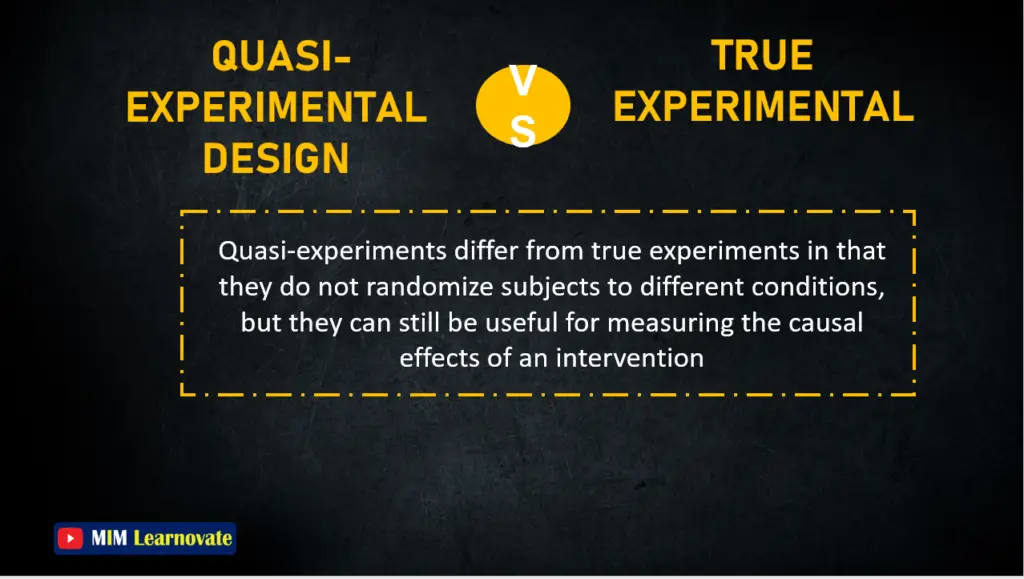
Features of Quasi-Experimental Design
A quasi-experimental design is a type of experimental design that is used to approximate true experimental designs. Quasi-experimental designs are typically used when true experimental designs are not possible or practical.
Read More: Sampling Bias in Probability Samples | Types, Examples | PPT
The key features of a quasi-experimental design include:
Presence of an independent variable
In a quasi-experimental design, there is an independent variable that is manipulated by the researcher. This independent variable is what allows the researcher to test for causality.
Read more: T-test | Example, Formula | When to Use a T-test
Absence of random assignment
In a quasi-experimental design, participants are not randomly assigned to conditions. This means that there may be confounding variables that could impact the results of the study.
Use of control groups
Control groups are used in quasi-experimental designs to control for confounding variables.

Types of Quasi-Experimental Design
There are a few different types of quasi-experimental designs, which are described in more detail below.
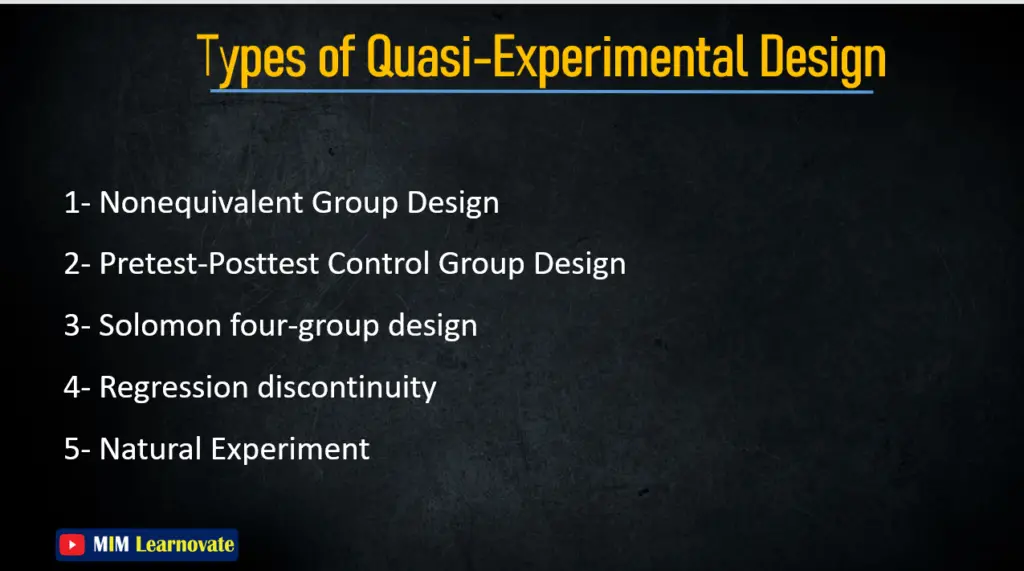
1- Nonequivalent Group Design
The nonequivalent group design is the most popular type of quasi-experimental design. In this design, the researcher does not randomly assign subjects to treatment and control groups. Instead, the researcher assigns subjects to groups based on some preexisting characteristic, such as gender or grade level.
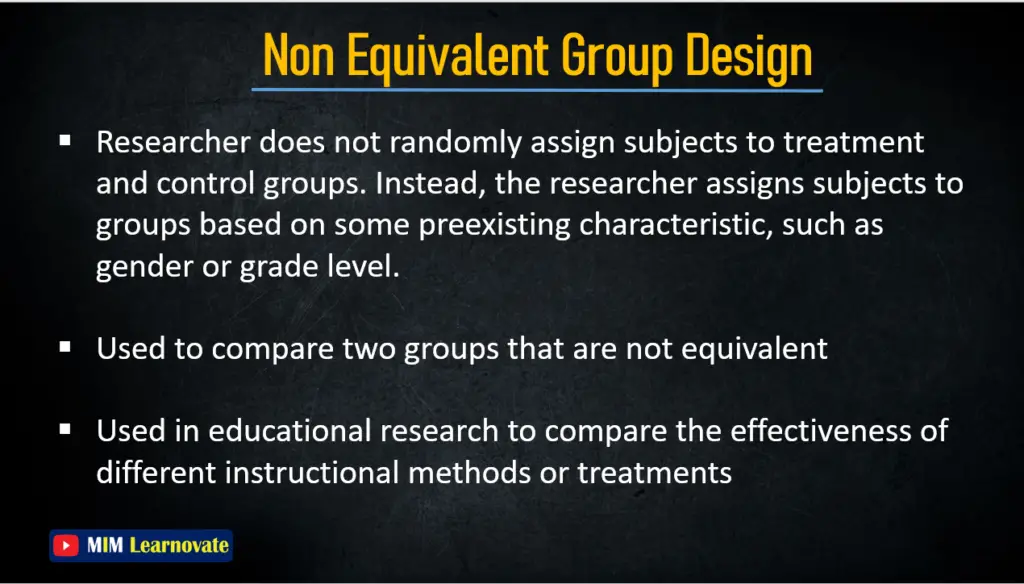
The nonequivalent group design is a research method used to compare two groups that are not equivalent. This type of design is often used in educational research to compare the effectiveness of different instructional methods or treatments.
Advantages of Nonequivalent Group Design
There are several advantages to using a nonequivalent control group design.
- less expensive and time-consuming than other designs
- easier to recruit participants for this type of study
- less likely to be confounded by extraneous variables than other designs
Disadvantages of Nonequivalent Group Design
There are also some disadvantages to using a nonequivalent control group design.
- can be difficult to ensure that the two groups are truly nonequivalent.
- this design does not allow for randomization of participants, which could lead to selection bias.

Example of Nonequivalent Group Design
An example of a nonequivalent groups design would be a study comparing the academic achievement of students in two different schools.
The first school might be an inner-city school with a high poverty rate and the second school might be a suburban school with a low poverty rate.
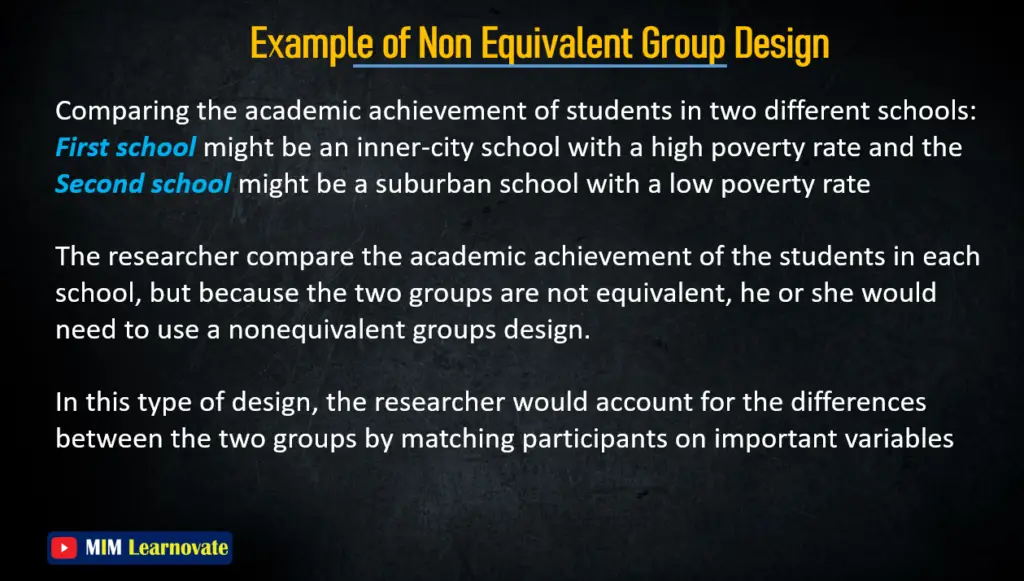
The researcher would want to compare the academic achievement of the students in each school, but because the two groups are not equivalent, he or she would need to use a nonequivalent groups design.
In this type of design, the researcher would need to account for the differences between the two groups by matching participants on important variables.
Read More: Chi-Square Test (Χ²) || Examples, Types, and Assumptions
2- Pretest-Posttest Control Group Design
The pretest-posttest control group design is similar to the nonequivalent control group design, but includes a pretest and a posttest. The pretest measures the dependent variable before any treatment has been applied.
A pretest-posttest control group design is an experiment in which there is a control group that does not receive the treatment and a separate group that does receive the treatment.

The groups are compared on a posttest, which measures the outcome variable. This design is used to study Cause-and-effect relationships.
Advantage of Pretest-Posttest Control Group Design
- allows the researcher to see if there is a difference between the two groups on the outcome variable after controlling for any pre-existing differences.
Disadvantage of Pretest-Posttest Control Group Design
- can be expensive and time-consuming to administer both a pretest and posttest.
- this design does not allow the researcher to make any inferences about causality.

Example of Pretest-Posttest Control Group Design
One group of students might be taught using traditional methods while another group is taught using more innovative methods. The researcher would then compare the scores on a posttest to see if there was any difference in learning.
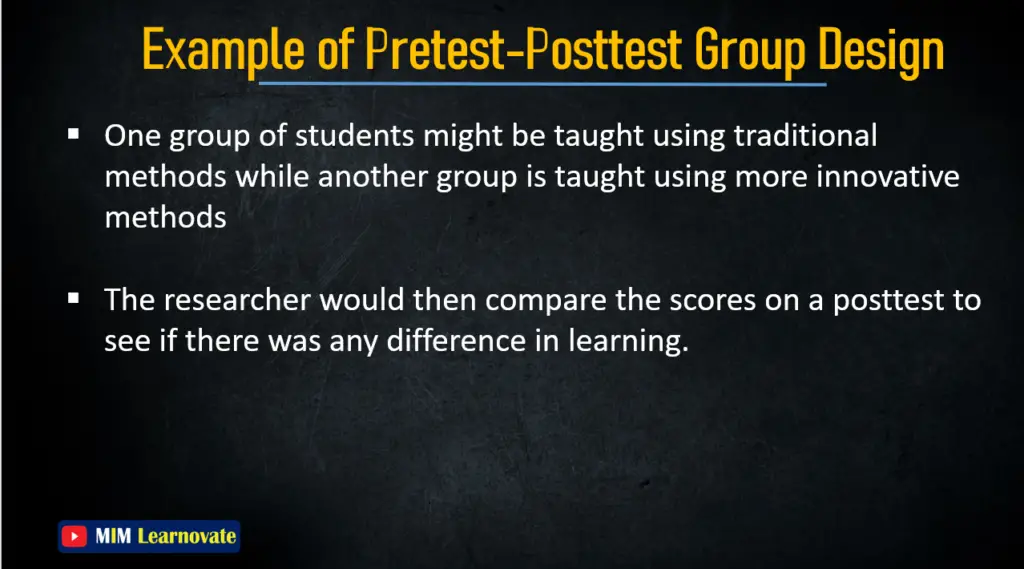
3- Solomon four-group design
This type of design is named after its inventor, Dr. Paul Solomon. He developed this method in the 1960s as a way to improve upon existing methods for studying the effects of treatments.
In a Solomon four-group design, there are four groups of participants, each of which experiences a different condition.
A Solomon four-group design is a type of experimental design that is used to study the effects of treatments on study participants.

This design is used when there are four groups of participants, and each group receives a different treatment. The results of the study can then be analyzed to see if there are any significant differences between the groups.
This design is used to compare two treatments by using a control group and a placebo group.
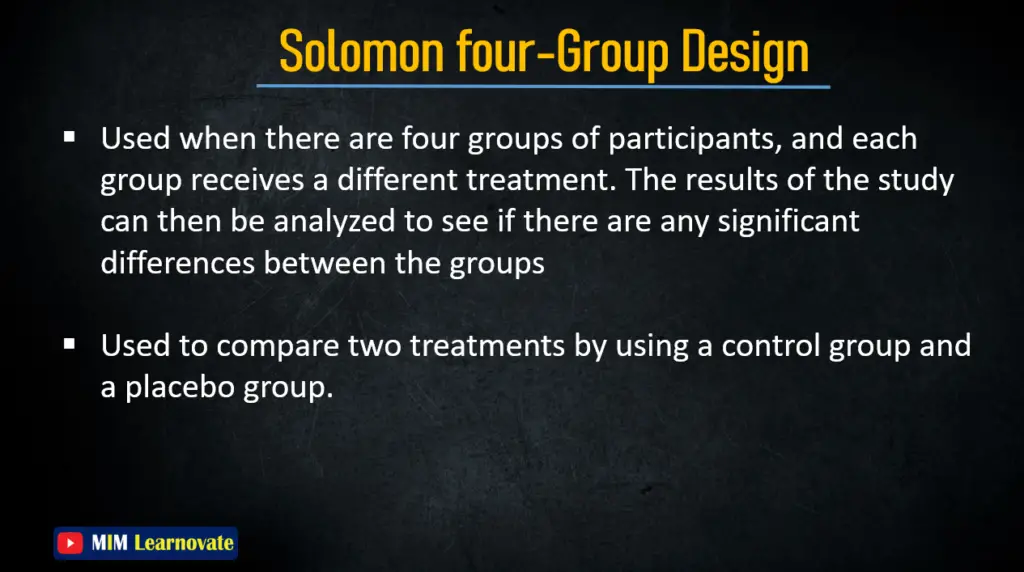
Advantage of Solomon four-group design
- minimizes the amount of variability between groups.
Disadvantage of Solomon four-group design
- requires a large number of participants in order to be effective.
This can make it difficult to find enough people who are willing to take part in the study.

4- Regression discontinuity
Regression discontinuity occurs when the value of a dependent variable changes abruptly as the value of an independent variable passes through a specified point. This point is known as the breakpoint. The breakpoint can be either an explicit value or a virtual value that is based on a mathematical formula.
Regression discontinuity can be caused by many factors, including changes in policy, changes in technology, and changes in market conditions. It is important to identify regression discontinuity in order to properly interpret data and make decisions about future course of action.

A regression discontinuity occurs when a predictor variable is not randomly assigned, but there is a threshold that determines who does and does not receive the treatment.
This non-random assignment creates two groups: those who are just above the threshold (the treated group) and those who are just below the threshold (the control group). The difference in outcomes between these two groups can then be attributed to the treatment.
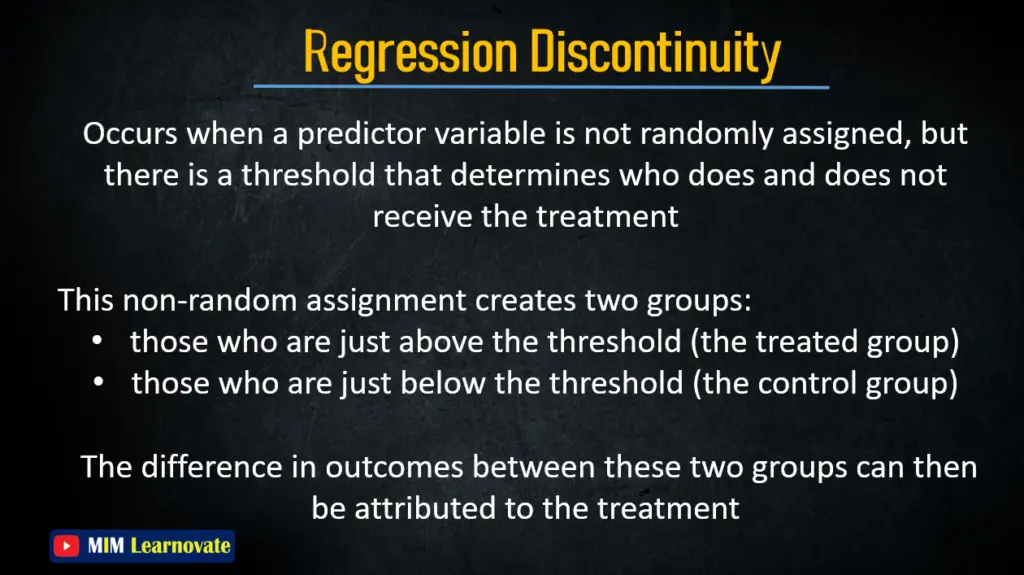
Example of Regression discontinuity
Consider a program that provides tutoring to students who are just below the cutoff for passing their grade level.
- students who receive tutoring (the treated group) will likely see an increase in their grades
- the students who do not receive tutoring (the control group) will likely see a decrease in their grades.
The difference in these outcomes can be attributed to the program itself.

Types of regression discontinuity
There are two types of regression discontinuity:
- Positive regression discontinuity
- Negative regression discontinuity
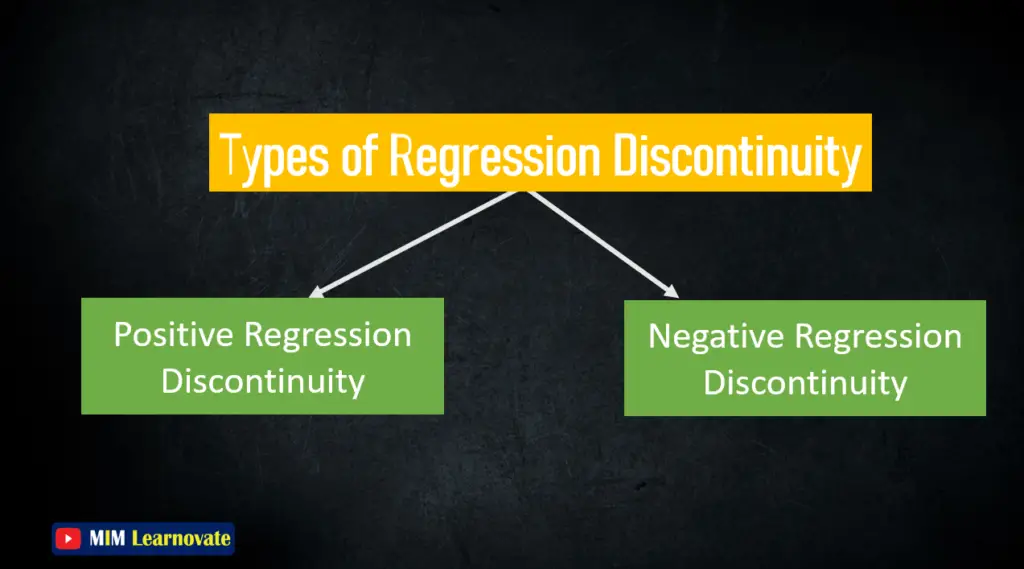
Positive Regression Discontinuity
Positive regression discontinuity occurs when the dependent variable increases as the independent variable passes through the breakpoint.
Negative Regression Discontinuity
Negative regression discontinuity occurs when the dependent variable decreases as the independent variable passes through the breakpoint.

5- Natural Experiment
A natural experiment is a quasi-experiment that occurs when circumstances allow for an observational study to be conducted under conditions that approximate a controlled experiment.
Natural experiments are often used to study situations in which it would be unethical or impractical to conduct a controlled experiment, such as when investigating the long-term effects of exposure to a naturally occurring toxin.

Natural experiments are not true experiments because the investigator does not manipulate the exposure of interest and cannot randomize subjects to different groups.
However, natural experiments can provide useful information about causal relationships. When well-designed, natural experiments can yield results that are similar to those of randomized controlled trials.
There are several factors that must be considered when designing a natural experiment, including:
- selection bias
- confounding factors
- exposure misclassification
Natural experiments are often criticized for their lack of control over these important variables.
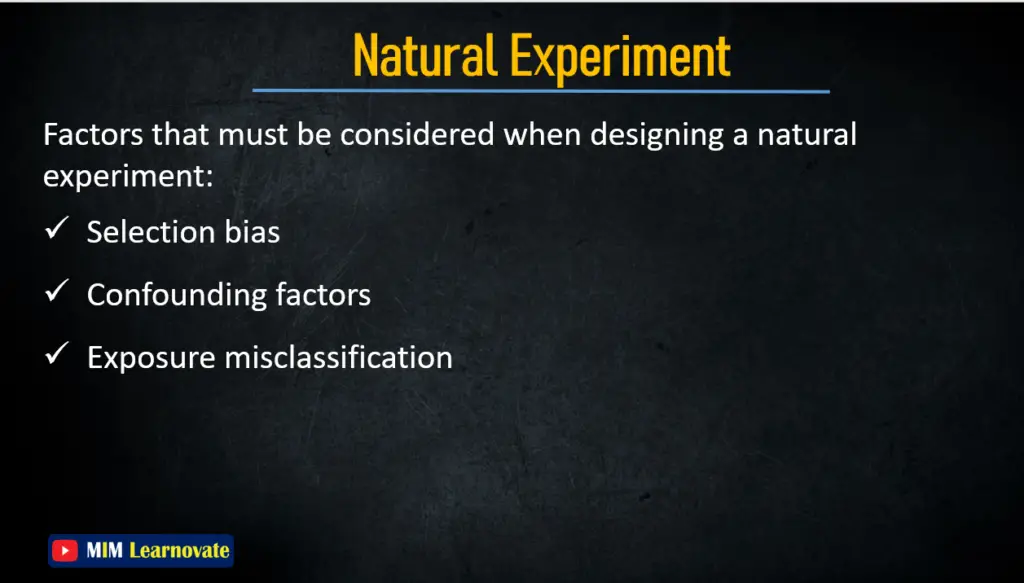
Example of Natural Experiment
One well-known example of a natural experiment is the case of lead poisoning in children living in inner-city housing.
Lead paint was commonly used in homes built before 1978, and children living in these homes are at risk for exposure to lead if the paint begins to deteriorate. In 1992, the U.S. government banned the use of lead-based paints, providing a natural experiment to study the effects of lead exposure on children’s health.
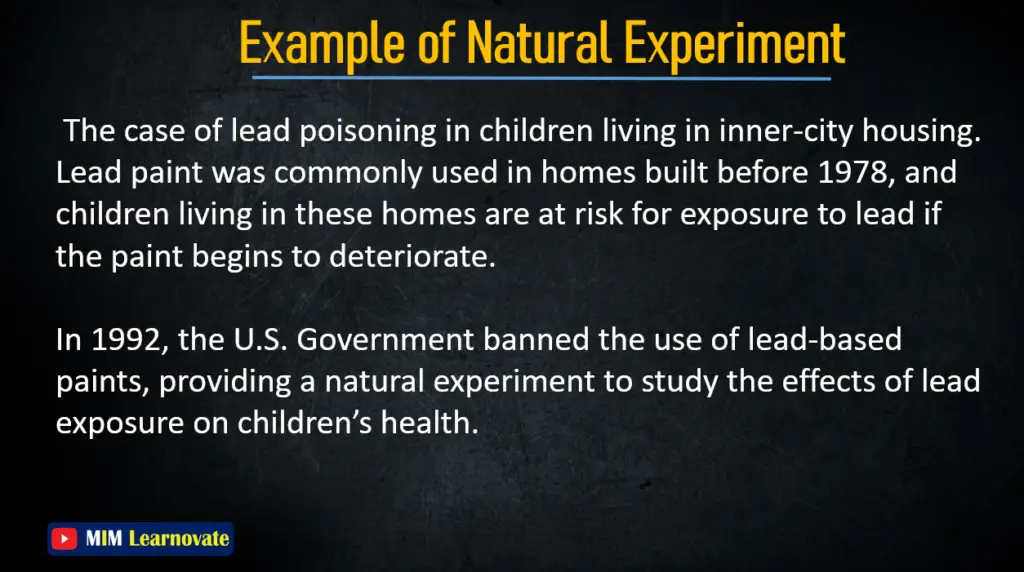
Uses of Quasi-Experimental Design
In social science research, a quasi-experimental design is often used This type of design
- when it is not possible or ethical to conduct a true experiment.
- used to study cause-and-effect relationships.
In a quasi-experiment, the researcher manipulates one or more independent variables and measures the dependent variable to determine if there is a causal relationship between the two.
For example, if you want to study the effects of smoking on health, you can’t randomly assign people to smoke or not smoke. However, you could compare health outcomes of people who do smoke with those who don’t.
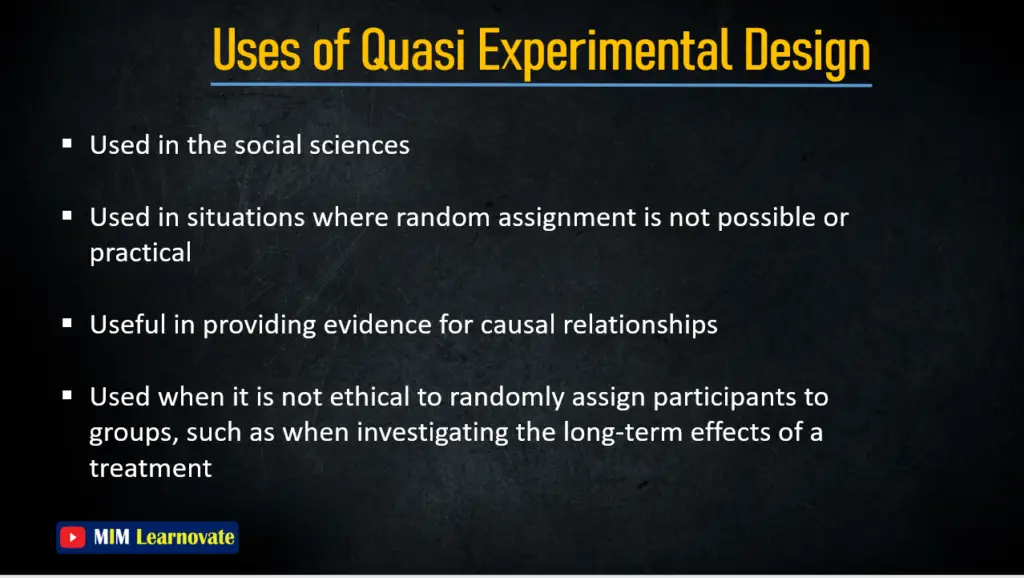
Pros of Quasi-Experimental Design
Quasi-experimental design has several advantages over other research designs.
- it can be used to study naturally occurring phenomena in real-world settings.
- allows researchers to control for extraneous variables that might confound the results of an experiment.
- Designing quasi-experiments can be ideal for determining what is best for the population. Also known as external validity.
- used to study rare events or populations that would be difficult or impossible to study using a traditional experimental design.
Because quasi-experimental designs often use existing data, they can be used to study rare events or populations that would be difficult or impossible to study using a traditional experimental design.
- can provide more information about causality than correlational designs.

Cons of Quasi-Experimental Design
There are a few cons to using quasi-experimental design when conducting research.
- rely on observational data, which can be less reliable than data collected through controlled experiments.
- It offers less internal validity than true experiments.
- It may allow the researcher’s personal bias to be taken into account.
- It does not use randomly assigned groups so it is difficult to determine if the results are due to the intervention or other factors.

Other articles
Please read through some of our other articles with examples and explanations if you’d like to learn more about research methodology.
Statistics
- PLS-SEM model
- Principal Components Analysis
- Multivariate Analysis
- Friedman Test
- Chi-Square Test (Χ²)
- T-test
- SPSS
- Effect Size
- Critical Values in Statistics
- Statistical Analysis
- Calculate the Sample Size for Randomized Controlled Trials
- Covariate in Statistics
- Avoid Common Mistakes in Statistics
- Standard Deviation
- Derivatives & Formulas
- Build a PLS-SEM model using AMOS
- Principal Components Analysis using SPSS
- Statistical Tools
- Type I vs Type II error
- Descriptive and Inferential Statistics
- Microsoft Excel and SPSS
- One-tailed and Two-tailed Test
- Parametric and Non-Parametric Test
Methodology
- Research Methodology Quiz MCQ
- Research Methods
- Quantitative Research
- Qualitative Research
- Case Study Research
- Survey Research
- Conclusive Research
- Descriptive Research
- Cross-Sectional Research
- Theoretical Framework
- Conceptual Framework
- Triangulation
- Grounded Theory
- Quasi-Experimental Design
- Mixed Method
- Correlational Research
- Randomized Controlled Trial
- Stratified Sampling
- Ethnography
- Ghost Authorship
- Secondary Data Collection
- Primary Data Collection
- Ex-Post-Facto
Research
- Table of Contents
- Dissertation Topic
- Synopsis
- Thesis Statement
- Research Proposal
- Research Questions
- Research Problem
- Research Gap
- Types of Research Gaps
- Variables
- Operationalization of Variables
- Literature Review
- Research Hypothesis
- Questionnaire
- Abstract
- Validity
- Reliability
- Measurement of Scale
- Sampling Techniques
- Acknowledgements
2 Comments
I really value your piece of work, Great post.
To the mimlearnovate.com administrator, Nice post!